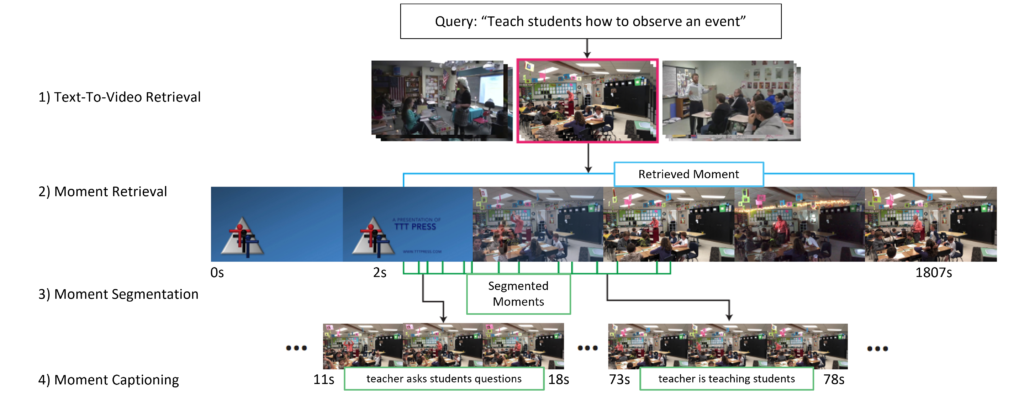
Co-PI Bansal and his team have been investigating the performance of vision-and-language models on classroom videos. Specifically, the team has focused on text-to-video retrieval, video-moment retrieval, video-moment segmentation, and video-moment captioning models, which retrieve the most relevant video from a set of candidate videos according to an input text query and caption the small step/moment-based contents of the video. Since current vision-and-language models often do not focus on education, this work was an opportunity to examine how well existing models can perform on educational videos with zero-shot and few-shot learning.
For text-to-video retrieval, the team evaluated CLIP ViTB/32 and a CLIP that is fine-tuned on the HODINI dataset developed in Bansal’s lab. For moment retrieval, moment segmentation, and moment captioning, the team has focused on evaluating a HODINI unified model. A collection of 26 classroom videos from YouTube were utilized for this work. OpenCV was used to extract frames from the videos at a rate of 1 frame per second. Captions for all the videos were collected via manual annotation. Results from experiments indicated that current models, such as CLIP and HODINI, are able to perform reasonably well on all four of tasks (text-to-video retrieval and moment retrieval/segmentation/captioning) in this domain. However, there is still significant room for improvement, especially for text-to-video retrieval and moment captioning. From the analysis, it was also found that audio is a critical component of understanding these videos (indicated by low CLIP performance on moment retrieval and low SSIM performance on moment segmentation).
Upcoming Plans: Although initial zero-shot and fine-tuning results show significant promise, the videos used in this work cover a broad range of teaching styles and topics, so fine-tuning the model on a larger more diverse set of videos/annotations could prove helpful. Increasing the detail of the moment-captioning annotations in a larger dataset could also help, as currently generated captions are short and undetailed. Finally, exploring the application of the vision-and-language models to videos of student collaborative learning in the Institute’s AI-driven narrative-centered learning environments will be an important direction.